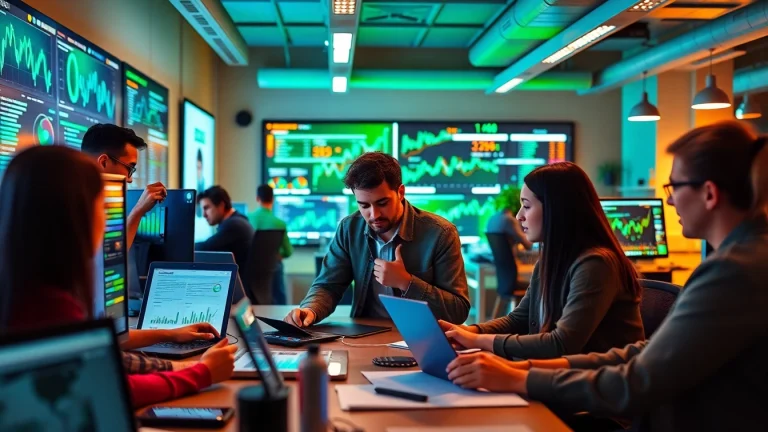
Unlocking Insights: How informaticsview.com Elevates Your Understanding of Data Analytics
Understanding Informatics and Its Significance
What is Informatics?
Informatics can be defined as the science of processing data for storage and retrieval. It encompasses a range of disciplines that investigate the role of information in various contexts, including health, business, and education. By leveraging computing technologies, informatics aids in managing, analyzing, and transforming data into insightful information that can drive decision-making processes.
The Role of Informatics in Modern Society
In today’s digital age, the role of informatics is increasingly pivotal. It helps organizations streamline operations, enhances communication, and enables data-driven decisions that optimize performance. For instance, healthcare informatics has led to better patient outcomes by improving diagnosis and treatment options through data integration. Moreover, businesses utilize informatics to analyze consumer behavior, refine marketing strategies, and maintain competitive advantages. As such, informatics is not just a tool; it is a cornerstone of contemporary society, influencing how we live, work, and interact.
Key Terminology in Informatics
Understanding informatics also requires familiarity with its foundational terminologies. Some of the essential terms include:
- Data: Raw facts that can be processed to extract information.
- Information: Processed data that has meaning in a specific context.
- Knowledge: Insights gained from understanding information in context.
- Big Data: Data sets that are so complex and voluminous that traditional data processing software cannot manage them.
- Data Governance: The management of data availability, usability, integrity, and security in an enterprise.
Data Analytics: The Core of Informaticsview.com
Types of Data Analytics Explained
Data analytics is at the heart of informatics, providing the means to transform raw data into actionable insights. There are several types of data analytics:
- Descriptive Analytics: This type focuses on summarizing historical data to understand what has happened in the past. It uses statistical methods to analyze trends and patterns.
- Diagnostic Analytics: Going a step further, diagnostic analytics seeks to explain why something happened by identifying causes and correlating patterns in data.
- Predictive Analytics: This advanced approach involves using algorithms and machine learning techniques to forecast future trends based on historical data.
- Prescriptive Analytics: The most complex form, prescriptive analytics recommends actions to achieve desired outcomes, leveraging optimization and simulation algorithms.
Tools and Technologies Used
The use of sophisticated tools and technologies is essential in the realm of data analytics. Some widely used tools include:
- Tableau: A powerful visualization tool that enables users to create interactive and shareable dashboards.
- R and Python: Programming languages commonly used for statistical analysis and data manipulation.
- SAS: A software suite developed for advanced analytics, business intelligence, and data management.
- Power BI: A business analytics tool from Microsoft that provides interactive visualizations and real-time insights.
- SQL: Structured Query Language is essential for querying databases to extract meaningful data.
Case Studies from informaticsview.com
To illustrate the impact of data analytics, several case studies from informaticsview.com offer practical examples. For instance:
- Healthcare Improvement: A hospital utilized predictive analytics to reduce patient wait times by analyzing historical admission data and streamlining resource allocation.
- Retail Optimization: A retail chain employed descriptive analytics to identify best-selling products and optimize inventory management, resulting in increased sales and reduced markdowns.
- Financial Risk Assessment: A financial institution implemented diagnostic analytics to detect fraudulent transactions, saving millions through swift action on flagged activities.
Challenges in Data Handling and Solutions
Common Data Management Issues
Despite its benefits, data management presents various challenges, including:
- Data Quality: Poor quality data leads to inaccurate analyses and can misinform decision-making.
- Data Silos: Isolated data systems within organizations can prevent comprehensive analytics.
- Regulatory Compliance: Keeping up with regulations like GDPR can be challenging for businesses handling personal data.
- Scalability: As data volumes increase, ensuring that systems can scale to handle more data without loss of performance is crucial.
How Informaticsview.com Addresses These Challenges
In response to these challenges, informaticsview.com provides resources and insights that help organizations overcome common data-related issues:
- Educational Content: The website offers articles and tutorials on best practices for data cleansing and quality improvement.
- Interoperability Guidelines: Providing strategies to break down silos between data systems, increasing data accessibility across departments.
- Compliance Frameworks: Informing businesses about data protection laws and offering templates for compliance documentation.
- Scalability Solutions: Highlighting cloud-based technologies designed to dynamically adjust to changing data loads.
Best Practices for Data Governance
Implementing robust data governance practices is essential for maximizing the value of analytics while minimizing risks. Key practices include:
- Data Stewardship: Assigning roles and responsibilities for data management across the organization.
- Data Quality Protocols: Establishing standards for data entry, validation, and analysis to enhance quality and reliability.
- Access Controls: Defining who can access and use different types of data to ensure security and privacy.
- Continuous Training: Investing in ongoing education for employees about data governance and best practices.
Future Trends in Informatics and Data Analytics
Emerging Technologies Shaping the Field
The future of informatics is heavily influenced by emerging technologies such as:
- Artificial Intelligence (AI): AI is transforming analytics by automating data processing and enabling complex predictive models. Machine learning algorithms learn from data patterns, evolving with minimal human intervention.
- Cloud Computing: The shift towards cloud solutions allows for greater scalability and flexibility in data storage and analytics applications, enhancing accessibility and collaboration.
- Blockchain Technology: Immutability and transparency offered by blockchain can revolutionize data security and integrity, particularly in sectors like finance and healthcare.
Potential Industry Changes and Impacts
The integration of these technologies is likely to lead organizations to rethink their analytics strategies. For example:
- Companies may adopt a more decentralized approach to data management, leveraging cloud platforms to ensure accessibility across global teams.
- Decision-making processes in businesses may become increasingly automated, using AI to predict trends and make recommendations based on real-time data.
- The emphasis on ethical data usage will likely rise, with organizations focusing on maintaining consumer trust through transparency and compliance with regulations.
Preparing for the Future with informaticsview.com
To stay ahead in this rapidly evolving landscape, organizations can utilize resources from informaticsview.com to forecast upcoming trends and adjust their strategies accordingly. By embracing continuous learning and adopting innovative tools, businesses can navigate the complexities of data analytics effectively.
Getting Started with Data Analytics
Beginner Tools and Resources from informaticsview.com
For those new to data analytics, several beginner-friendly tools and resources are available at informaticsview.com. These may include:
- Excel: A well-known tool that provides excellent entry-level analytics capabilities with various functions for data manipulation and visualization.
- Google Analytics: A web analytics service that provides insights into website traffic and user behavior.
- Online Courses: Platforms offering courses on data analytics, data science fundamentals, and specific software usage.
Steps to Conduct Your First Analysis
Getting started in data analytics involves several key steps:
- Define Your Objective: Clearly state what you want to achieve with the analysis. Are you attempting to discover trends, make predictions, or describe the current state?
- Collect Data: Gather the necessary data from relevant sources, ensuring its quality and relevance to your analysis.
- Prepare the Data: Clean and preprocess the data for analysis, converting it into a format suitable for the tools you are using.
- Analyze the Data: Use your chosen analytical tools to derive insights from the data.
- Interpret Results: Draw conclusions from your analysis, understanding the implications of your findings.
- Communicate Findings: Present your insights in an understandable manner, using visualizations where appropriate.
Evaluating Success Metrics in Data Projects
Measuring the success of your data analytics projects is vital for continuous improvement. Key metrics may include:
- Accuracy: The degree to which your predictions or conclusions match actual outcomes.
- Return on Investment (ROI): Gauging the financial return on data projects compared to their costs.
- User Engagement: For web analytics, metrics such as bounce rate and session duration can indicate the effectiveness of content.
- Decision Impact: Analyzing how insights from data analytics have influenced and improved organizational decisions.