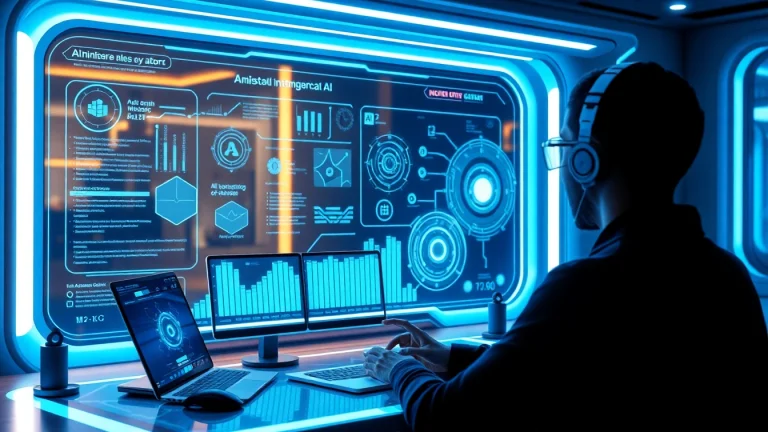
Understanding Agentic AI: The Future of Autonomous Decision Making
Introduction to Agentic AI
In the rapidly evolving landscape of artificial intelligence, a new category of AI has emerged – Agentic AI. This advanced technology represents a significant leap from traditional AI models, focusing not only on data interpretation but also on autonomous decision-making. As organizations strive for efficiency and innovation, understanding the nuances of Agentic AI becomes essential to grasp its implications for the future of work and technology.
Definition and Key Features
Agentic AI refers to systems that possess the ability to act autonomously toward achieving specific goals without continual human guidance. Unlike traditional AI, which primarily follows explicit programming and user commands, Agentic AI can interpret data, make decisions based on complex criteria, and even learn from outcomes to improve future performance. Key features of Agentic AI include:
- Autonomy: The capacity to operate independently and make decisions without human intervention.
- Adaptability: The ability to learn from past encounters, adjust to new data, and refine processes in real-time.
- Complex Problem-Solving: Competences in addressing complex, multi-step problems that require iterative reasoning.
Comparison to Traditional AI Systems
Traditional AI systems rely heavily on pre-defined algorithms and datasets to perform tasks. These systems are excellent in executing repetitive tasks and analyzing large volumes of data but often lack the ability to operate under uncertainty or adapt dynamically. In contrast, Agentic AI enhances functionality by enabling:
- Decision-Making: Agentic AI can evaluate options and choose the best course of action based on contextual factors.
- Strategic Planning: These systems can generate plans that evolve as environments change, making them ideal for scenarios requiring flexibility.
- Real-World Applications: Agentic AI’s decision-making prowess allows for applications across diverse sectors, from autonomous driving to supply chain optimization.
Relevance in Today’s Technology Landscape
With businesses increasingly seeking efficiency and reliability, the relevance of Agentic AI has surged. Its capabilities align well with current industry demands for automated decision-making processes. Furthermore, as organizations face increasingly complex operational landscapes influenced by global events and technological advancements, Agentic AI offers a way to navigate these challenges effectively. As such, it commands attention in discussions surrounding digital transformation and future readiness.
Core Technologies Behind Agentic AI
Machine Learning and Decision Making
At the heart of Agentic AI lies machine learning (ML), specifically algorithms that enable systems to learn from data inputs and outcomes. These algorithms are structured to discern patterns and correlations within data, subsequently utilizing these insights in decision-making. The process typically involves:
- Data Input: Feeding the system extensive datasets which represent varied scenarios and outcomes.
- Learning Phase: The AI processes the data, identifying trends and patterns to enhance its decision-making algorithms.
- Decision Execution: Once trained, the AI can autonomously make decisions based on analysis performed on live data.
Data Adaptability and Autonomy
Agentic AI systems excel due to their ability to adapt their knowledge and processes continuously. This adaptability allows them to manage unpredictable situations more effectively than traditional AI. With sophisticated learning techniques, they can:
- Adjust to New Information: Unlike static models, they can amend their decisions and strategies based on real-time data inputs.
- Contextual Analysis: The system analyzes context, allowing for more relevant decision-making that aligns with current conditions or user needs.
Real-Time Problem Solving
Agentic AI’s real-time problem-solving capabilities enable it to address issues as they arise, employing reasoning processes that mimic human-like judgment while drawing on vast data resources. This dynamic problem-solving ability positions Agentic AI as an invaluable asset in sectors where timing is critical, such as finance, healthcare, and logistics. Its mechanisms include:
- Event Recognition: Identifying changes or anomalies within operating environments that may necessitate immediate action.
- Solution Generation: Formulating actionable plans based on the recognized issues, leveraging decision-making frameworks that are pre-trained on extensive datasets.
- Outcome Evaluation: Assessing the outcomes of executed solutions to refine future responses.
Applications of Agentic AI
Industry Use Cases
Agentic AI is finding a plethora of applications across varied industries, showcasing its versatility and effectiveness. Some notable use cases include:
- Healthcare: Implementing autonomous diagnostics systems that can analyze medical data to suggest treatments.
- Finance: Risk assessment models that autonomously evaluate market volatility and adjust investment strategies.
- Manufacturing: Robotic process automation systems that adapt production lines based on input from IoT devices.
- Customer Service: AI-driven chatbots capable of high-level interactions without requiring human oversight.
Benefits for Businesses
Integrating Agentic AI into business operations presents several benefits, including:
- Increased Efficiency: Reducing the time taken for decision-making processes and elevating operational efficiency.
- Cost Reduction: Automating routine tasks frees up human resources for higher-level strategic endeavors.
- Enhanced Accuracy: Reducing human error in data interpretation and decision-making processes by relying on advanced analytics.
Examples from Leading Companies
Numerous organizations are leveraging Agentic AI to transform their operations. For instance:
- Amazon: Utilizes intelligent agents in its logistics and supply chain management to monitor and adjust shipment schedules in real-time.
- Google: Implements Agentic AI in its search algorithms, improving result relevancy without significant human intervention.
- IBM: By using Watson, IBM provides autonomous AI solutions that support various enterprise needs from customer service to data analytics.
Challenges and Considerations
Ethical Implications of Autonomous Systems
The rise of Agentic AI raises several ethical questions surrounding autonomy and decision-making. Concerns revolve around accountability in scenarios where AI systems operate independently, leading to:
- Decision Accountability: Defining who is responsible when an Agentic AI system makes a detrimental decision.
- Bias in Decision-Making: Ensuring that data used for training AI does not perpetuate biases or inequality.
Security and Data Privacy Issues
As organizations adopt Agentic AI, they face security challenges, particularly with data privacy. The collection and analysis of personal data can lead to:
- Data Breaches: Increased vulnerability to cyber attacks as systems store and process vast amounts of sensitive information.
- Regulatory Compliance: Adhering to laws regarding data protection, such as GDPR, becomes crucial for companies operating in multiple jurisdictions.
Managing Human-AI Collaboration
With the integration of Agentic AI into workplaces, fostering a collaborative environment between human workers and AI systems is crucial. Challenges include:
- Cultural Shift: Organizations need to nurture a work culture that embraces AI’s role as a partner rather than a replacement.
- Training and Education: Up-skilling employees to effectively collaborate and utilize AI technology enhances the collective potential.
The Future of Agentic AI
Predictions for Emerging Technologies
Looking ahead, Agentic AI is poised to integrate with various emerging technologies such as IoT and blockchain, leading to even more sophisticated applications. For instance, in healthcare, real-time data from wearable devices paired with Agentic AI can improve patient outcomes through timely interventions.
Integration with Existing Systems
As organizations adopt Agentic AI, the focus will also turn toward integrating these systems with legacy solutions. This strategy often involves:
- API Development: Facilitating communication between new AI platforms and existing systems through Application Programming Interfaces.
- Gradual Implementation: Testing Agentic AI alongside traditional systems ensures operational continuity while transitioning towards full integration.
Long-Term Impact on Industries
The evolution of Agentic AI is set to redefine industries over the long term. Its autonomous capabilities will likely lead to:
- New Business Models: Companies may shift toward service models that rely on AI for core functionalities, changing the competitive landscape.
- Restructured Workforce: Labor markets will adapt as demand shifts from routine tasks to roles focused on oversight and strategy.