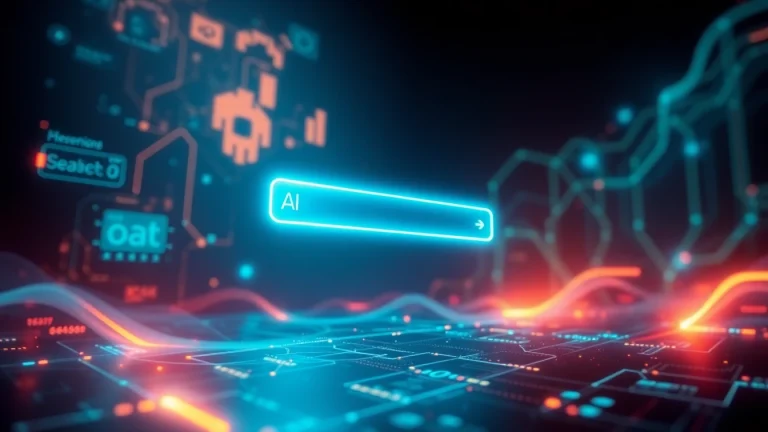
Reimagining the Future: How AI Search Engines Are Transforming Online Navigation
Understanding AI Search Engines
Definition and Functionality
AI search engines represent a groundbreaking evolution in how users access information online. Unlike traditional search engines that primarily rely on keyword matching and links to rank content, AI search engines leverage artificial intelligence to understand user intent and deliver more relevant results. By utilizing advanced algorithms and machine learning techniques, these engines can sift through vast amounts of data, extracting valuable information and presenting it in a user-friendly format. For instance, when a user inputs a query, an AI search engine can analyze the context of the request, compare it against a broader dataset, and filter results accordingly, ensuring precision and efficiency in information retrieval.
Key Features of AI Search Engines
- Natural Language Processing (NLP): AI search engines utilize NLP to understand and process human language, making it easier for users to enter queries in a conversational format.
- Contextual Understanding: These engines can consider the context of previous searches or even the specific needs of the user, enhancing the relevance of the results.
- Personalization: AI search engines can analyze user behavior over time to tailor search results to individual preferences, making the search experience more efficient.
- Real-Time Data Access: Many AI-driven platforms can pull from live data sources and databases, presenting users with the most current information available.
- Visual and Multimedia Search: In addition to text-based queries, AI search engines are increasingly adept at processing visual and audio content, enabling users to conduct searches via images or voice recognition.
Comparison with Traditional Search Engines
Traditional search engines, like Google, primarily operate on predetermined algorithms that rank pages based on keywords, backlinks, and other SEO factors. While effective in many scenarios, this approach can sometimes fall short of delivering nuanced results, particularly for complex queries.
In contrast, AI search engines focus more on semantic understanding and contextual comprehension. For example, if a user asks about “the best restaurants in New York,” a traditional engine might return a list based on keyword frequency and backlinks. An AI search engine, however, can assess user reviews, current trends, geographical data, and even social media feedback to curate a list that genuinely reflects user preferences and real-time conditions.
Benefits of AI Search Engines
Improved Search Accuracy
One of the most significant advantages of AI search engines is their ability to provide improved search accuracy. Through advanced algorithms and machine learning, these engines can grasp user intentions and deliver results that are more aligned with what the user is genuinely seeking. This capability leads to more relevant outcomes, reducing the time spent searching through irrelevant information.
Enhanced User Experience
The user experience is significantly augmented by the interactive capabilities of AI search engines. These platforms often include features like voice search, image recognition, and chat-based interfaces, allowing users to engage in a more natural and intuitive manner. Furthermore, the ability to provide immediate responses and rich snippets enhances engagement and satisfaction.
Real-Time Data Utilization
AI search engines excel in leveraging real-time data, fetching the most current information available from various sources. This is particularly useful in fast-paced industries like finance or news, where the latest updates can significantly affect decision-making processes. Users benefit by receiving answers pertinent to the current context, ensuring their insights and actions are based on the most accurate information.
Challenges and Limitations
Data Privacy Concerns
As with any technology that involves data processing, AI search engines face challenges related to data privacy. User data is often required to enhance personalization and algorithm accuracy, leading to concerns over how this information is collected, used, and protected. Companies operating AI search engines must implement stringent measures to ensure user data is handled responsibly and in compliance with regulations such as GDPR.
Algorithmic Bias Issues
Another significant challenge is the potential for algorithmic bias, which can arise from the data used to train AI models. If the training data reflects societal biases, the AI may unintentionally produce skewed or discriminatory results. This issue necessitates continuous evaluation and refinement of AI models to ensure fairness and inclusiveness in the information provided.
Dependence on Internet Connectivity
AI search engines often require a stable internet connection to function effectively. This dependence can pose challenges in areas with inadequate internet access, limiting the availability of AI-driven information retrieval for users in remote or underserved locations. As such, infrastructural improvements are essential to enable widespread access to these advanced tools.
Implementing AI Search Engine Solutions
Best Practices for Businesses
For businesses looking to leverage AI search engines, adopting the following best practices is crucial:
- Conduct Thorough Research: Understand the specific needs and behaviors of your audience to tailor search solutions that genuinely meet their demands.
- Integrate with Existing Systems: Ensure that the AI search engine can be seamlessly incorporated into existing workflows and databases to enhance efficiency and usability.
- Focus on User Education: Educate users about how to utilize AI search functionalities effectively, including understanding query inputs and interpreting results.
- Continuously Optimize: Regularly monitor and analyze search results to identify areas for improvement in terms of accuracy and relevance.
Integrating AI Search Engines into Existing Systems
Integrating AI search engines into current systems requires a strategic approach. Organizations should assess existing data architectures, ensuring that AI tools are compatible and can draw from relevant data sources effectively. Collaboration between technical teams and end-users is essential to ensure that the integration process meets practical needs and doesn’t disrupt existing workflows.
Measuring Success and Performance Metrics
To gauge the effectiveness of an AI search engine implementation, businesses should focus on a range of performance metrics:
- Search Accuracy: Measure how effectively the AI engine returns relevant results based on user queries.
- User Engagement: Track user interaction with search results, including click-through rates and time spent on pages.
- User Satisfaction: Utilize surveys and feedback mechanisms to assess user satisfaction with the search experience.
- Response Time: Monitor how quickly the AI engine returns results to users, as speed is crucial for user satisfaction.
Future Trends in AI Search Engines
Predictions for AI Advancements
The future of AI search engines is poised for exciting advancements, including improvements to machine learning algorithms that will enhance their accuracy and efficiency. Expect to see the proliferation of multi-modal search capabilities, allowing users to interact with these engines through varied formats, including voice, text, and images, thus broadening the scope of how information can be accessed.
Potential Impact on SEO Practices
The rise of AI search engines is likely to transform SEO practices significantly. As AI-driven platforms prioritize content relevance and quality over traditional SEO metrics, businesses will need to adapt their strategies to focus on creating high-quality, informative content that addresses users’ needs. Additionally, as AI becomes adept at understanding context, the emphasis will shift towards optimizing for user intent rather than merely keyword usage.
The Role of User Feedback in AI Evolution
User feedback will play an increasingly critical role in the evolution of AI search engines. Engaging users in the development process—by soliciting their insights and experiences—will allow developers to fine-tune AI algorithms better and create search environments that cater closely to user preferences and behaviors. By fostering a feedback loop, AI search engines can continuously evolve to meet the changing landscape of user expectations.