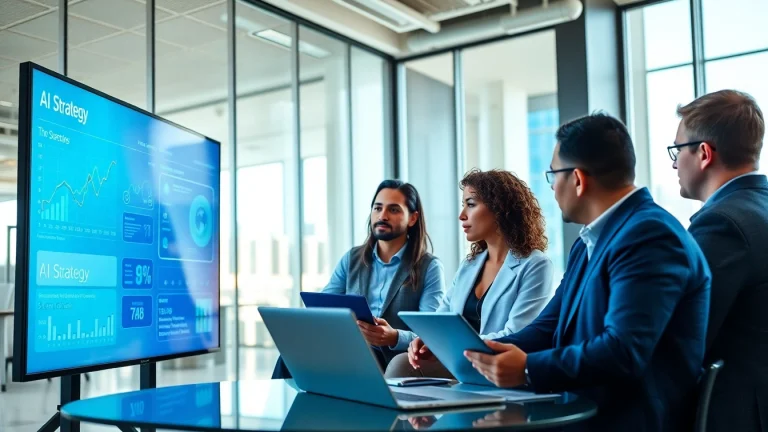
Building an Effective AI Strategy: Key Components and Best Practices
Understanding AI Strategy Essentials
Defining AI Strategy and Its Importance
In the rapidly evolving landscape of technology, an ai strategy has become a pivotal component for organizations aiming to harness the power of artificial intelligence effectively. An AI strategy is essentially a comprehensive framework that delineates how an organization can leverage AI technologies to enhance its operational efficiency, decision-making process, and overall competitive advantage. As businesses navigate this digital transformation journey, the importance of a well-thought-out AI strategy cannot be overstated. It serves as a roadmap guiding enterprises through complex AI deployments while ensuring alignment with their overarching business objectives.
Common Challenges in Developing AI Strategies
While the potential benefits of implementing an AI strategy are vast, organizations often encounter several challenges during the development phase. One significant hurdle is the lack of clear understanding of AI technologies and their capabilities. Many decision-makers may not be equipped with the necessary knowledge to identify applicable use cases within their organization, leading to skepticism or misalignment of potential initiatives. Another prominent challenge is data-related issues, including data quality, accessibility, and the integration of disparate data sources. Without high-quality data, the effectiveness of AI models can diminish significantly. Additionally, organizations may struggle with aligning their AI initiatives with existing business processes, resulting in resistance from employees and stakeholders. Finally, ethical considerations and regulatory compliance can pose significant barriers in establishing a responsible AI strategy.
Core Components of a Successful AI Strategy
A successful AI strategy is built on several core components that ensure a structured approach to implementation. These components include:
- Leadership Commitment: Executive support is crucial for driving AI initiatives. Leaders need to advocate for AI integration and allocate the necessary resources.
- Clear Objectives: Defining clear, measurable objectives helps organizations understand the specific outcomes they wish to achieve through AI adoption.
- Data Strategy: A solid data strategy involves clearly defined processes for data collection, storage, processing, and analysis, ensuring that the data is reliable and accessible.
- Technology Infrastructure: Organizations need to assess and invest in the right technologies and tools to support AI applications.
- Talent Acquisition: Hiring or developing talent with the requisite skills in data science, machine learning, and AI is vital for the success of AI projects.
- Ethical Guidelines: Establishing ethical guidelines ensures that AI implementations are fair, transparent, and compliant with regulations.
Aligning AI Strategy with Business Goals
Integrating AI with Your Organizational Vision
To maximize the impact of an AI strategy, businesses must align it closely with their organizational vision. This involves understanding how AI can address specific business challenges and contribute to strategic goals. Organizations should start by conducting a thorough analysis of current operations to identify inefficiencies and areas where AI can add value. For example, a manufacturing company might deploy AI to optimize supply chain logistics, thus reducing costs and improving delivery times. Creating cross-functional teams that include members from various departments can help foster a holistic approach, facilitating the identification of AI opportunities that align with business priorities.
Identifying Key Performance Indicators (KPIs)
Defining KPIs is essential for evaluating the success of AI initiatives. KPIs should be directly linked to business objectives and measurable in a way that reflects AI impacts. Common AI-related KPIs include:
- Time to Market: The duration it takes to implement AI solutions and start receiving benefits.
- Cost Savings: Financial savings derived from enhanced operational efficiencies.
- Accuracy and Precision: The performance metrics of algorithms or models deployed within AI systems.
- Customer Satisfaction: Metrics assessing user experience improvements as a direct result of AI technology.
Strategic Use Cases for Your AI Strategy
Identifying strategic use cases is a critical step in implementing an effective AI strategy. Use cases should reflect areas where AI can provide significant value and drive competitive advantage. Some examples of impactful AI use cases include:
- Predictive Analytics: Leveraging historical data to predict future trends, enabling proactive decision-making.
- Chatbots and Virtual Assistants: Enhancing customer engagement and support through AI-driven conversational interfaces.
- Fraud Detection: Utilizing machine learning algorithms to detect and prevent fraudulent activities in real-time.
- Personalization: Tailoring offerings based on customer behavior and preferences to improve satisfaction and retention.
Implementation Steps for a Robust AI Strategy
Building Your AI Team: Roles and Responsibilities
Developing a skilled team is fundamental to the success of any AI initiative. Organizations must clarify roles and responsibilities to ensure the smooth implementation of their AI strategy. Key roles may include:
- Data Scientists: Professionals who analyze data and build algorithms to provide insights and predictions.
- Data Engineers: Individuals responsible for developing data pipelines and infrastructure to ensure data quality and availability.
- AI/ML Engineers: Specialists who focus on building, testing, and deploying machine learning models and AI systems.
- Business Analysts: Team members who translate business requirements into technical specifications and vice versa.
Selecting Appropriate Technology and Tools
Choosing the right tools and technologies is critical for successful AI implementation. Organizations should evaluate their current technology stack and determine what additions or upgrades are necessary for supporting AI initiatives. Common technology considerations include data storage solutions, machine learning frameworks, and cloud computing resources. Organizations may opt for open-source tools, commercial solutions, or a hybrid approach depending on their specific needs and budgetary constraints.
Phased Implementation for Effective Results
Rather than attempting a full-scale implementation of AI across the organization at once, a phased approach offers a more manageable and effective route. This approach allows organizations to test and refine their AI strategies in smaller segments. A recommended implementation phase might include:
- Pilot Projects: Undertaking small-scale projects to test AI applications and gather insights.
- Iterative Development: Continuously improving projects based on feedback and performance metrics.
- Scaling Solutions: Gradually expanding successful AI applications across departments and functions.
Best Practices in AI Strategy Deployment
Maintaining Data Privacy and Ethics in AI
Data privacy and ethical considerations are paramount in any AI strategy. Organizations must comply with regulations such as the GDPR and ensure responsible data usage. Establishing data governance frameworks is essential to maintain transparency, data quality, and user privacy. Furthermore, creating a culture that promotes ethical AI development can help in gaining stakeholder trust and support.
Continuous Education and Training for Your Workforce
The rapidly changing nature of AI technology necessitates ongoing education and training for staff members. Organizations should implement training programs that equip employees with the necessary skills to work effectively alongside AI systems. This not only enhances collaboration but also helps in dispelling fears regarding job security. Providing access to resources such as webinars, workshops, and online courses can foster a learning culture and encourage innovation.
Agility and Adaptation in AI Strategy
The technological landscape is always in flux; therefore, organizations must remain agile in their AI strategies. This involves regularly reviewing and adapting AI initiatives based on performance data, market changes, and emerging technologies. An agile approach allows organizations to pivot swiftly in response to new challenges and opportunities, thereby ensuring the longevity and relevance of their AI strategy.
Measuring the Success of Your AI Strategy
Essential Metrics to Assess AI Performance
Evaluating the success of AI initiatives requires a robust framework for performance measurement. Organizations should consider both quantitative and qualitative metrics to capture the full impact of their AI strategy. Some essential metrics include:
- Return on Investment (ROI): Comparing the financial benefits of AI implementations against their costs.
- Operational Efficiency Gains: Measuring time saved, resource efficiency, and productivity improvements.
- User Adoption Rates: Acknowledging how readily employees and customers embrace AI solutions.
- Customer Feedback: Analyzing qualitative feedback to assess customer satisfaction regarding AI interactions.
Feedback Loop: Iterating Your AI Strategy
Establishing a feedback loop is critical for the ongoing refinement of an AI strategy. Organizations should actively solicit feedback from users and stakeholders to identify areas for improvement. Conducting regular reviews of AI performance based on established KPIs aids in making data-driven adjustments. This iterative process ensures that the AI strategy remains aligned with business objectives while accommodating changing operational or market conditions.
Case Studies of Successful AI Strategy Implementations
Examining real-world examples of organizations that have successfully implemented AI strategies can provide valuable insights and inspire others to embark on their AI journeys. For instance:
- Amazon: Amazon utilizes AI for personalized product recommendations, enhancing the shopping experience while increasing sales.
- Netflix: Through sophisticated algorithms, Netflix analyzes viewer behavior to offer tailored content suggestions, driving user engagement.
- IBM: The IBM Watson platform has been deployed in healthcare settings to assist doctors with diagnostics and treatment recommendations, demonstrating AI’s potential to revolutionize industry practices.