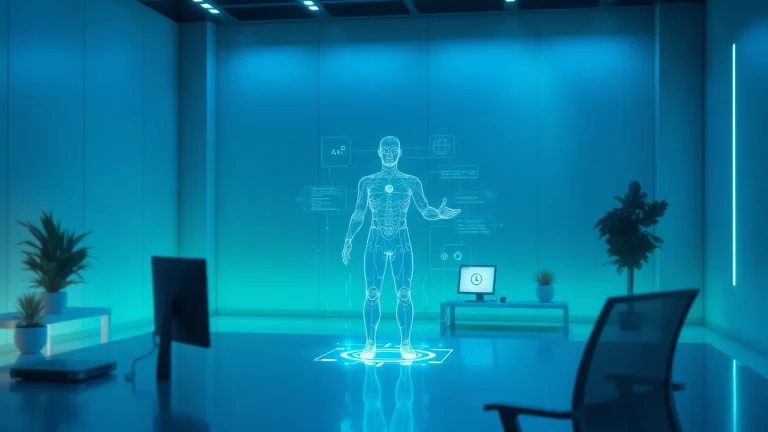
Understanding Agentic AI: An Autonomous Future for Intelligent Decision Making
What is Agentic AI?
Defining the Concept
Agentic AI refers to a subset of artificial intelligence systems that possess the capability to execute tasks autonomously, making decisions and taking actions without direct human intervention. Unlike traditional AI systems that require constant supervision and input, agentic AI is designed to pursue complex goals, solve problems, and adapt its strategies based on its environment. It leverages sophisticated reasoning and iterative planning to navigate intricate scenarios, often applying these capabilities across various industries and applications.
At its core, agentic AI embodies the potential for machines to operate with a degree of independence that revolutionizes how we interact with technology. These systems not only analyze patterns and data but also take decisive actions that can lead to significant outcomes. The landscape of agentic AI is rapidly evolving, attracting interest from businesses aiming to enhance operational efficiency and productivity. For a deeper exploration of its applications and implications, consider visiting Agentic AI.
Key Characteristics
The characteristics that define agentic AI include:
- Autonomy: The ability to operate independently and make decisions without continuous human oversight.
- Adaptability: The capacity to adjust behaviors and strategies based on new information or changes in the environment.
- Goal-Oriented Functionality: Designed to achieve specific objectives, ensuring actions are aligned with defined outcomes.
- Learning Ability: Utilizes machine learning techniques to improve performance through experience and data analysis.
- Complex Problem Solving: Employs advanced algorithms to navigate multifaceted challenges that may vary in complexity.
Real-life Applications
Agentic AI finds its application in a variety of fields, showcasing its versatility and effectiveness. Some notable examples include:
- Healthcare: Automated systems that assist in diagnosis and treatment planning for patients, substantially reducing time and errors.
- Finance: Investment algorithms that autonomously analyze market trends and execute trades based on predictive analytics.
- Manufacturing: Robotics that oversee production lines, adjusting workflows in real-time to optimize output and reduce waste.
- Customer Service: Intelligent chatbots capable of handling customer inquiries and guiding them through complex processes without human assistance.
- Transportation: Self-driving vehicles that make real-time decisions to navigate traffic and ensure passenger safety.
Differences Between Agentic AI and Generative AI
Core Functionalities
Understanding the distinction between agentic AI and generative AI is crucial for grasping their respective functionalities and applications. While agentic AI focuses on decision-making and actions, generative AI centers around creating content. Below are key differences:
- Function: Agentic AI acts, while generative AI creates.
- Data Dependency: Agentic AI often relies on external data streams to inform its decisions, whereas generative AI generates output based on internal models trained on datasets.
- User Interaction: Agentic AI systems may function with minimal user input, whereas generative AI typically requires user prompts to generate relevant content.
Use Cases and Examples
Examples of agentic AI in practice include:
- Chatbots: Automating customer service by understanding and responding to inquiries without human intervention.
- Autonomous Drones: Managing logistics and delivery routes effectively by adapting in real-time to changing conditions.
Generative AI, on the other hand, is utilized for tasks such as:
- Content Creation: Generating written articles, graphic designs, or music based on user input.
- Art Generation: Creating visual art by learning patterns from a wide array of examples provided by artists.
Advantages and Limitations
Each type of AI presents its own advantages and drawbacks. For agentic AI, some of the advantages include:
- Increased Efficiency: Reduced need for human oversight can lead to faster outcomes and lower operational costs.
- Scalability: Easily scalable to handle large data sets and decisions across various sectors.
However, agentic AI also faces limitations:
- Complexity of Implementation: Integrating agentic AI within existing workflows can pose significant challenges.
- Lack of Understanding: These systems can sometimes operate in black boxes, making their decision processes opaque to users.
In contrast, generative AI provides unique advantages, such as creativity and generation of diverse outputs, but limitations exist, including reliance on quality input data to produce meaningful results.
Implementing Agentic AI in Businesses
Steps for Integration
To successfully integrate agentic AI within a business, several key steps should be undertaken:
- Assessment of Needs: Identify areas where autonomous decision-making can improve efficiency.
- Technology Evaluation: Research and select appropriate AI systems and platforms that align with business objectives.
- Pilot Program: Implement a pilot project to test the effectiveness of agentic AI in a specific area.
- Training and Support: Ensure staff is trained on new systems and provide ongoing technical support.
- Feedback and Iteration: Gather feedback from users to optimize and iterate on the AI solutions.
Challenges to Overcome
Implementing agentic AI is not without its hurdles. Common challenges include:
- Resistance to Change: Employees may resist adopting AI technologies due to fear of job displacement or distrust in AI capabilities.
- Data Privacy Concerns: The handling of personal data and AI decision-making can raise ethical concerns.
- Integration Complexities: Merging new technologies with legacy systems can be technically challenging and resource-intensive.
Best Practices for Success
To ensure successful deployment of agentic AI, consider the following best practices:
- Engagement with Stakeholders: Involve affected parties early in the process to increase buy-in and acceptance.
- Ethical Guidelines: Develop clear ethical guidelines for AI usage that address concerns about bias and transparency.
- Iterative Development: Use an agile approach to continuously improve and adapt AI systems based on user experience and feedback.
The Future of Agentic AI
Trends to Watch
As technology advanced, several trends are emerging within the realm of agentic AI that businesses should monitor:
- Increased Collaboration with Humans: Enhanced interaction models may allow agentic AI systems and humans to work in tandem more effectively.
- Improved Natural Language Processing: Developments in NLP will allow agentic AI to communicate more intuitively with users.
- Ethical AI Development: A growing focus on ethical considerations surrounding AI usage will lead to more transparent and accountable systems.
Potential Impacts on Workforce
The rise of agentic AI will likely have significant implications for the job market:
- Job Transformation: Many roles will shift from manual tasks to oversight and strategic positions, requiring reskilling.
- New Job Creation: New roles centered around designing, managing, and maintaining AI systems will emerge.
- Enhanced Productivity: Teams may achieve greater output and efficiency, leading to growth opportunities.
Evolution of AI Technologies
The future will continue to see advancements in AI methodologies, including:
- Hybrid AI Models: Combining different AI approaches to create systems that leverage the strengths of each.
- Explainable AI: Development of algorithms that are transparent and can be easily interpreted by users.
- Federated Learning: AI systems that can learn from decentralized data sources, improving privacy and data security.
Case Studies of Successful Agentic AI Usage
Leading Companies Utilizing Agentic AI
Several organizations have successfully integrated agentic AI, yielding impressive results:
- NVIDIA: Through its AI reasoning technologies, NVIDIA is enhancing decision-making processes across various industries, including automotive and healthcare.
- Amazon: Utilizes agentic AI in its fulfillment centers to optimize logistics and inventory management, leading to faster delivery times and reduced costs.
- IBM: Implemented agentic AI in various applications to drive efficiency and predict customer behavior in retail.
Results and Performance Metrics
Companies implementing agentic AI often report measurable improvements, including:
- Cost Savings: Streamlined operations have led to reductions in labor costs and increased profitability.
- Efficiency Gains: Companies report significant time savings in processes previously handled by humans.
- Enhanced Customer Satisfaction: Improved service delivery times and responsiveness to customer needs.
Lessons Learned from Implementation
Businesses that have integrated agentic AI provide valuable insights:
- Address Ethical Considerations: Prioritize ethical implications and develop guidelines to govern AI usage.
- Invest in Training: The workforce must be equipped with skills to thrive alongside AI technologies.
- Continuous Monitoring: Establish processes for monitoring and optimizing AI systems to ensure they meet evolving business needs.